Key Strategies for Driving Engagement with AI Chatbots
AI chatbots can’t just exist in your customer journey—they need to enhance it. And that only happens when there’s strategic intent behind implementation. Below are five proven strategies that leading enterprises use to drive real engagement (and measurable ROI) with AI chatbots.
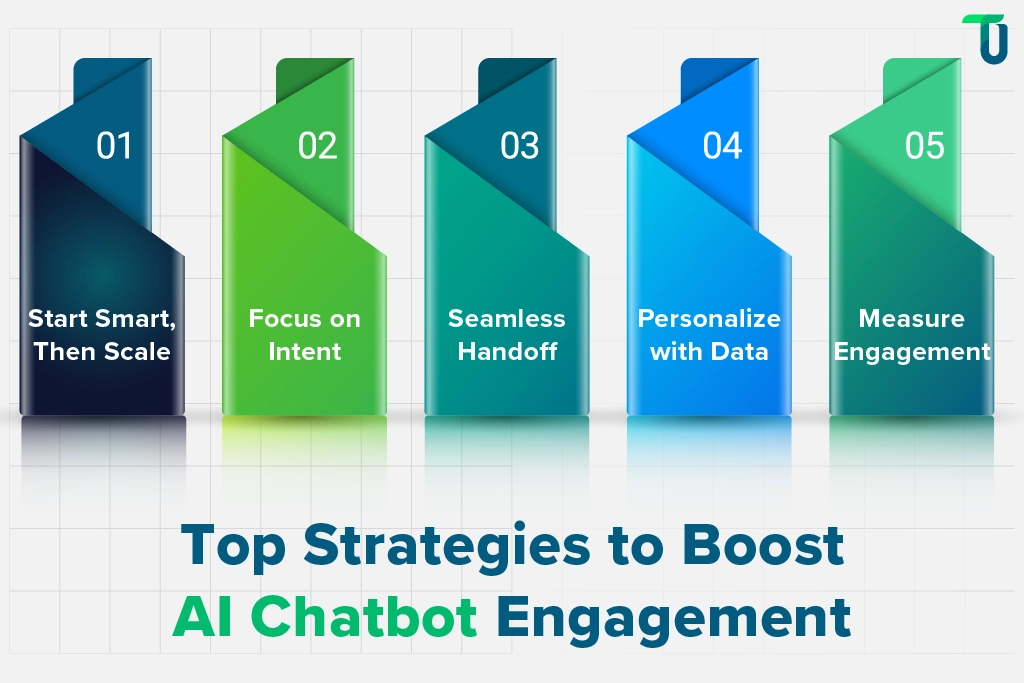
1. Start With the Right Use Case—Then Scale
One of the biggest missteps in chatbot deployment? Trying to do everything at once. The smarter move: start small, solve a specific problem, and expand based on validated outcomes.
Real-life example:
Babylon Health, a leading digital-first healthcare provider, started with a medical AI chatbot to triage basic symptoms and guide patients to the right care path. Over time, it evolved into a full-fledged virtual assistant integrated with remote consultation services, reducing primary care strain and supporting over 10 million consultations globally.
What to do:
Start by identifying one high-volume, repetitive customer touchpoint (e.g., order tracking, appointment scheduling, prescription refills), then build from there using conversational data.
2. Design for Intent, Not Just Interaction
An effective AI chatbot doesn't just “chat”—it solves. That requires understanding customer intent and mapping responses to outcomes that matter.
For AI chatbots in eCommerce, this could mean recognizing whether a customer is browsing, comparing, or ready to buy—and then nudging them with personalized product suggestions, promo codes, or restock alerts.
Pro Tip: Use NLP models trained on historical support and sales data to fine-tune your chatbot’s response logic and conversion triggers.
3. Human Handoff Should Feel Seamless
No matter how smart your AI gets, human intervention will always play a role—especially in high-stakes domains like healthcare or B2B enterprise support. The key is ensuring that the handoff from bot to human is frictionless and context-aware.
Case in point:
Lemonade: the AI-powered insurance company, uses chatbots to handle claims in under 3 minutes. But when cases become complex, the system escalates to human agents—armed with full chat history, policy details, and sentiment data. Result? Lightning-fast resolution and 4.9-star CSAT scores.
Implementation tip: Make sure your chatbot logs every interaction and passes that data cleanly into your CRM or helpdesk tools for a 360° view.
4. Personalize Every Interaction With Data
Generic responses don’t drive engagement. Data does. Leverage behavioral, transactional, and historical data to tailor every chatbot response to the individual user. In AI chatbot for customer service, this could mean preloading answers with the customer’s name, recent purchases, or support ticket history. For AI chatbots in healthcare, it could mean using EMR data (with consent) to deliver contextual advice and reminders.
5. Measure What Matters: Engagement, Not Just Deflection
A chatbot that deflects queries isn't necessarily one that engages. Track metrics that align with customer experience goals—like average resolution time, first contact resolution, conversion uplift, and customer sentiment—not just ticket deflection.
Real-world ROI:
H&M’s AI chatbot not only cut down support tickets by 60% but also increased product discovery by 3X through intelligent search and styling suggestions—directly driving sales and retention.
Action Step:Set up A/B tests to benchmark chatbot performance against traditional support and refine based on outcomes, not assumptions.
Let’s Redefine Customer Engagement—Together
Your customers expect real-time, personalized experiences. We’ll help you deliver them at scale, with AI that works.
Architecture That Powers Success: What CTOs Must Know
Behind every smart, scalable AI chatbot lies an intentional architecture—one that goes beyond a fancy interface and gets down to real engineering. For CTOs, understanding the tech stack isn't optional—it’s critical to unlocking speed, personalization, and enterprise-grade outcomes.
Here’s what you need to build and scale AI chatbots that don’t just respond—but drive results.
1. Core Tech Stack: NLP, ML, and LLMs
At the heart of every successful AI chatbot is a blend of NLP (Natural Language Processing), ML (Machine Learning), and increasingly, LLMs (Large Language Models) like OpenAI’s GPT, Google’s PaLM, or Meta’s LLaMA.
- NLP helps the bot understand human input—parsing intent, detecting sentiment, and extracting entities.
- ML personalizes the interaction—learning from past queries to improve responses over time.
- LLMs bring it all together—enabling deep, human-like conversations that go far beyond scripted replies.
Why it matters:
For use cases like medical AI chatbots, you need high-accuracy NLP for medical terminology and patient context. For AI chatbots in eCommerce, ML drives predictive suggestions based on user behavior and inventory data. Without this foundation, you’re left with a glorified keyword matcher.
2. Model Type: Rule-Based vs Hybrid vs Generative
Here’s the reality: no one-size-fits-all model works across your enterprise. The architecture you choose should reflect both the complexity of the queries and the maturity of your AI roadmap.
CTO takeaway: Start with hybrid if you’re early-stage. Move to generative when customer complexity—and expectations—scale. Just ensure you have strong fallback logic and human-in-the-loop safeguards in place.
3. Enterprise-Ready Integrations: CRM, Analytics & More
A chatbot can’t operate in isolation—it needs to plug into your existing enterprise systems to be truly effective.
- Integrate with CRMs like Salesforce or HubSpot to access customer history and tailor responses.
- Connect with analytics platforms to track behavior, drop-off points, and sentiment trends.
- Sync with enterprise apps—like ERPs, appointment scheduling tools, or patient portals—for contextual workflows.
Pro tip: Choose a chatbot platform that offers open APIs, pre-built integrations, and supports HIPAA/GDPR compliance if you’re operating in regulated sectors.
Measuring What Matters: KPIs and ROI Metrics
AI chatbots aren’t just shiny new tools—they’re performance engines. But without the right metrics, it’s impossible to separate hype from impact. If you’re serious about delivering business value, then it’s time to stop tracking vanity numbers and focus on KPIs that actually move the needle.
Let’s break down what the most forward-thinking leaders measure—and why it matters.
1. Engagement Benchmarks That Actually Tell a Story
It’s not just about how many people interact with your chatbot—it’s about how those interactions drive satisfaction, loyalty, and outcomes. These are the frontline KPIs that reveal whether your chatbot is creating value or friction:
- CSAT (Customer Satisfaction Score): Tells you how users feel immediately after interacting with the bot.
Target: 80%+ for tier-1 support.
- NPS (Net Promoter Score): Measures long-term sentiment—would they recommend your brand after using the bot?
Pro tip: Tie this to chatbot-specific journeys.
- Average Response Time: Should be sub-3 seconds for high-volume bots. Anything longer increases drop-offs.
- Resolution Rate: The real north star. Aim for 70–85% resolution without human intervention for AI chatbot for customer service deployments.
Example:
Sephora’s chatbot handles 11% of all customer service traffic with a 90% resolution rate—freeing up human agents and increasing CSAT by 20%.
2. Operational Efficiency: Proving the Bottom-Line Impact
C-suite leaders don’t just want happy users—they want efficiency gains. Here’s how the best-in-class organizations measure it:
- Cost-to-Serve Reduction: Calculate how much it costs to resolve a query via chatbot vs. human agent.
- On average, chatbots reduce support costs by 30% across industries.
- Agent Deflection Rate: Percentage of queries the bot handles without escalation.
- A well-trained medical AI chatbot can deflect up to 60% of low-acuity questions—cutting nurse triage workload
- FTE Savings: Convert bot-handled queries into full-time equivalent (FTE) hours saved.
3. Frameworks for Continuous Improvement
Even the best bots degrade over time if you’re not iterating. Treat your chatbot like a product—not a project. That means setting up feedback loops, performance reviews, and retraining cycles.
- Conversational Analytics: Audit drop-off points, common confusion triggers, and sentiment scores weekly.
- A/B Testing: Regularly test response formats, fallback logic, and proactive nudges.
- Training Data Updates: Feed your LLM or ML model with fresh conversations every 30–60 days.
Actionable Tip for CTOs: Deploy a closed-loop learning system—combine chatbot analytics with CRM and support ticket data to continually refine responses, improve accuracy, and boost business alignment.
Case Studies: How Industry Leaders Are Winning with AI Chatbots
Here's how leading organizations are leveraging AI chatbots to drive measurable business outcomes:
1. Vodafone: Enhancing Customer Experience with TOBi
Vodafone's AI-powered chatbot, TOBi, handles approximately 1 million conversations daily across 15+ markets. By integrating TOBi into platforms like WhatsApp, Vodafone achieved a 70% resolution rate for customer inquiries and reduced the cost per chat by 70%. Additionally, TOBi improved customer satisfaction, with Net Promoter Scores (NPS) nearing 80 for users interacting with the chatbot.
2. KLM Royal Dutch Airlines: Streamlining Customer Service with AI
KLM implemented an AI system powered by DigitalGenius to assist customer service agents in responding to social media inquiries. The AI suggests responses based on historical data, which agents can approve or modify, leading to faster and more consistent customer interactions. This hybrid approach allows KLM to handle a growing volume of social media queries efficiently while maintaining a personal touch.
3. Mayo Clinic: Reducing Patient Wait Times with AI Forecasting
Mayo Clinic utilized machine learning and demand forecasting to optimize appointment scheduling in its Neurology department. By analyzing various factors, including subspecialty demand and patient preferences, Mayo Clinic reduced patient wait times and improved the utilization of appointment slots, enhancing overall patient care.
4. Sephora
Sephora, the global beauty retailer, uses an AI chatbot on its website and messaging apps to deliver personalized product recommendations, book in-store appointments, and offer beauty tips. The result? A 11% increase in conversions and a 20% uptick in in-store bookings—without adding a single human agent.
AI chatbots don’t fail because of AI—they fail because of misalignment, bad data, or poor orchestration. If you get the foundations right—training data, escalation protocols, integrations, and compliance—chatbots can transform how your enterprise interacts with customers. Explore our blog on AI chatbot challenges with solutions to understand what usually goes wrong—and what smart leaders do to course-correct.
Future Outlook: AI Chatbots and the Autonomous Enterprise
AI chatbots are no longer just about answering FAQs or deflecting Tier-1 tickets—they’re becoming the frontline operators of the autonomous enterprise. We’re entering an era where bots don’t just respond. They anticipate. They transact. They learn. And they connect systems, people, and processes—without human push. Let’s unpack what’s next.
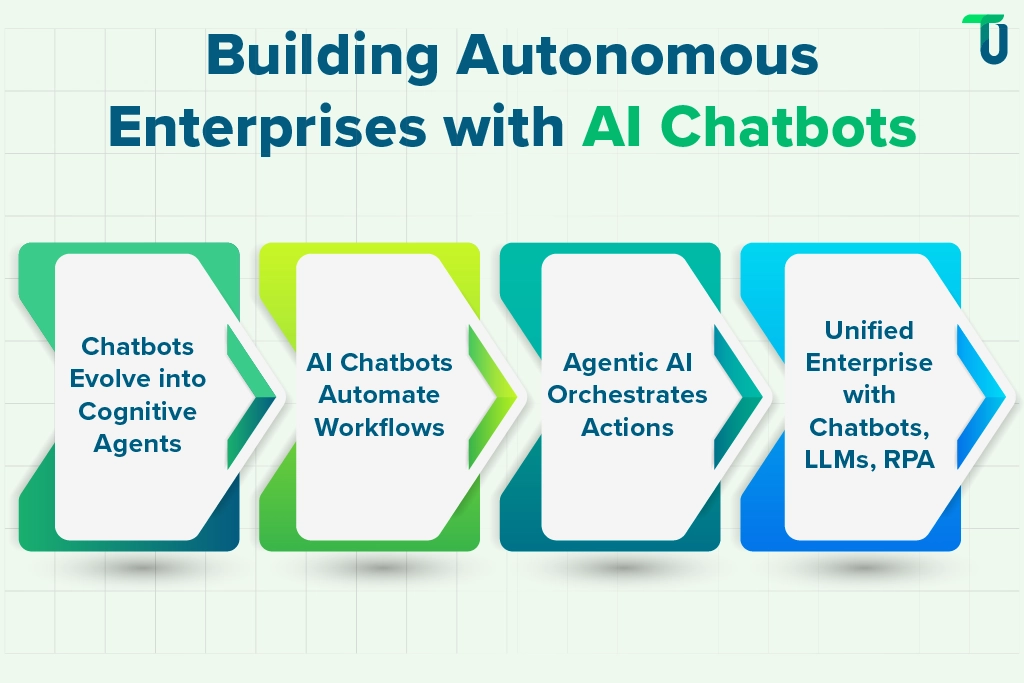
1. From Chatbots to Cognitive Agents
The next generation of chatbots will blur the line between virtual agents and digital employees. We’re talking about multi-modal, memory-driven AI systems that understand past interactions, interpret tone, and make contextual decisions.
Example: Imagine an AI chatbot for eCommerce that doesn’t just offer product recommendations—but factors in purchase history, returns, and even mood (via sentiment analysis) to proactively offer discounts or bundle deals.
This isn’t sci-fi—it’s already in pilot at retailers using Google Dialogflow CX and Emotion AI.
2. Self-Optimizing Workflows via AI Chatbots
In an autonomous enterprise, chatbots will not just talk—they’ll initiate actions across systems.
For example:
- A medical AI chatbot triages a patient based on symptoms,
- Schedules an appointment,
- Sends reminders,
- Flags potential risks to the doctor via EMR integration.
All in real-time. All without human intervention.
3. The Rise of Agentic AI: Orchestration, Not Just Interaction
In the near future, chatbots will operate like autonomous agents—navigating APIs, interpreting dashboards, placing orders, and triggering workflows across departments.
Example:
An AI chatbot for customer service might not just answer a delivery complaint—it could:
- Check warehouse stock,
- Notify logistics,
- Reissue an order,
- Email the customer a confirmation—all on its own.
This is the agentic AI movement—and it’s already being prototyped by OpenAI, LangChain, and Zapier AI.
4. Unified Enterprise Brain: Chatbots + LLMs + RPA
The future of chatbots lies in their fusion with large language models (LLMs) and robotic process automation (RPA). Together, they’ll form a digital nervous system for the enterprise—capable of decision-making, execution, and learning across the value chain.
Think of it like this:
- LLM = brain
- Chatbot = face
- RPA = hands
This triad will power everything from IT helpdesk to HR onboarding to supply chain forecasting—with zero human bottlenecks.
What CTOs and CIOs Should Prepare For?
- Build for interoperability now: Your chatbot should be composable, API-ready, and extensible..
- Invest in fine-tuning, not just deployment: LLMs are powerful, but contextual fine-tuning is key to enterprise value.
- Prioritize trust and explainability: As bots take on more decisions, governance will be non-negotiable.
Why TenUp Software Services Is Your Strategic Partner for AI Chatbot Success
Let’s be honest—building an AI chatbot that just “works” isn’t the hard part anymore. Building one that actually moves the business needle? That’s where TenUp Software Services wins.
At TenUp, we don’t just deploy bots—we engineer enterprise-grade AI solutions that are context-aware, secure, and deeply integrated with your existing systems and workflows.
From retail to healthcare, from BFSI to SaaS, we help global enterprises:
- Fine-tune chatbots with domain-specific intelligence
- Integrate with your CRM, ERP, and analytics stack—zero silos
- Apply LLMs, RPA, NLP, and hybrid models where they drive the highest ROI
- Ensure compliance, privacy, and governance from Day 1
And because we're not just technologists—we're AI transformation partners—we back every solution with a product mindset, agile delivery, and relentless optimization.
Whether you're launching your first AI chatbot for eCommerce, scaling a medical AI assistant, or automating customer service at enterprise scale—we make AI work for business, not just for demos. Let’s build the future of intelligent engagement together.
Don’t Let Human Bandwidth Limit Customer Delight
Stop relying on overloaded teams to deliver 24/7 support. Our Conversational AI scales empathy, without scaling cost. Automate Smarter Now.
Frequently asked questions
What is an AI chatbot for customer service?
An AI chatbot for customer service is a virtual assistant powered by artificial intelligence that instantly answers customer queries, automates repetitive tasks, and provides 24/7 support across chat, web, and messaging platforms—reducing wait times, boosting satisfaction, and routing complex issues to live agents when needed.
Can AI chatbots replace human customer service agents?
AI chatbots can automate routine queries and offer 24/7 support, but they can’t fully replace humans. Complex issues, emotional intelligence, and empathy still require human agents. A hybrid approach—AI for speed, humans for nuance—is the most effective customer service model.
What are the benefits of using AI chatbots for customer service?
AI chatbots offer 24/7 instant support, reduce response times, cut costs, and handle high volumes without fatigue. They ensure consistent answers, boost customer satisfaction, and free up human agents for complex tasks—making service faster, smarter, and scalable.
What industries use AI chatbots for customer service?
AI chatbots are widely used in e-commerce, banking, healthcare, telecom, travel, and SaaS industries to improve support and automate customer engagement.
How much does it cost to build an AI chatbot for customer service?
Basic AI chatbots can cost between $500–$5,000. Custom-built or enterprise-grade solutions may range from $10,000 to $100,000+, depending on features and integrations.
How can I train a custom AI chatbot using my own data?
You can train a custom AI chatbot by uploading your data sources, such as FAQs, product manuals, or support documents, into platforms like Landbot or Azure's QnA Maker, which utilize natural language processing to understand and respond to user inquiries.