What is AI Web Scraping?
AI-powered web scraping is the use of Artificial Intelligence (AI), Machine Learning (ML), and Natural Language Processing (NLP) to extract and process web data efficiently. Unlike traditional methods, AI-driven scrapers adapt to dynamic websites, interpret content contextually, and mimic human-like browsing for accurate and scalable data collection. This technology helps businesses and researchers gather high-quality insights while ensuring ethical and legal compliance.
The Evolution of Web Scraping: From Manual to AI-Driven
The origin of Web Scraping can be traced back to the birth of the World Wide Web in 1989. The first web robot (Wanderer), and the first crawler-based web search engine (JumpStation) were released in 1993. At this stage, web scraping was a manual process involving copying data from web pages and pasting it into local databases.
In the second stage of web scraping, data was harvested using simple scripts that parse static HTML pages and extract specific data elements. So, scripting and rule-based systems were built. Beautiful Soup was released in 2004 to pull data from HTML files. These methods worked well with static websites. However, when websites turned dynamic i.e. they changed content frequently and loaded it asynchronously, these methods were unable to adapt to their layout and complex navigation to extract data.
Enter stage three of web scraping. Sophisticated systems capable of navigating complex web architectures were developed. The first such system was Web Integration Platform version 6.0, which was launched in 2006 by Stefan Andresen. It highlighted necessary web page content and structured data in a usable format. It was a visual web scraping software and the foundation for web scraping as we know it now.
Using APIs and RSS feeds, consent-based data scrapers were developed. The advent of Big Data led to the development of web scrapers capable of handling large datasets and enabling data analytics. Integrating Machine Learning (ML) algorithms in web scraping tools increased their capabilities multi-fold in handling complex websites.
This journey brings us to the current stage of web scraping that utilizes advanced AI technologies. We’ll discuss this stage in detail in the next section.
How AI Transforms Web Scraping
Advanced AI technologies are taking web scraping to new heights. With Natural Language Processing (NLP), Vision AI, and Deep Learning, web scrapers can better understand the context and interpret content. It leads to better data extraction outcomes. To counteract these intelligence scrapers, new and more complex methods to prevent scraping are being used. This is inspiring the development of new techniques to overcome legal and ethical challenges. Here’s how AI is benefiting web scraping processes and outcomes:
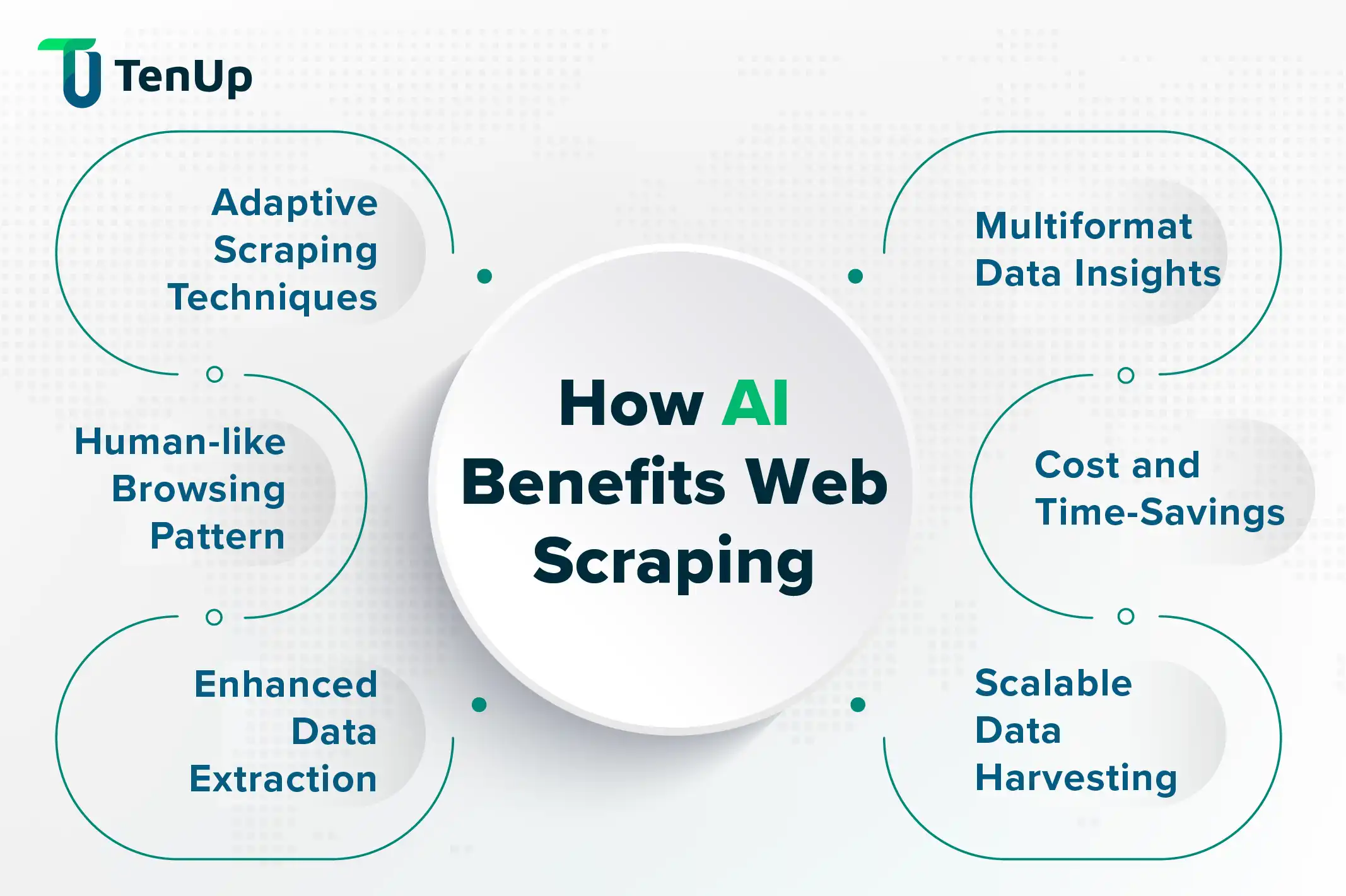
Adaptive Scraping Techniques: Navigating dynamic and complex websites is a key challenge for web scrapers. AI-enabled website scraping solutions using Deep Learning models do not rely on patterns in the layout and structure of the websites. Instead, they parse data more efficiently and understand the context of the content to extract relevant information. They also make relevant decisions while scraping websites to improve the quality of data harvested.
For example, if a business requires competitor product information, this scraper will identify a product name on the website even if its location and format change frequently.
Human-like Browsing Pattern: Websites implement anti-scraping measures to prevent automated data mining. They detect the repetitive pattern of traditional scrapers and block them. Gen AI models can be trained to mimic human behavior- their actions and even timing. Using reinforcement learning algorithms, their navigation patterns can be optimized to appear more natural. This reduces their risk of detection for successful data collection.
For example, AI-based scrapers showcase human-like behavior and blend in with genuine user traffic to avoid detection.
Enhanced Data Extraction Outcomes: Utilizing NLP techniques, scrapers can be trained to not just collect data but also clean and structure it in a usable format. Returning to our example of gathering competitor product information, NLP-powered scraping solutions identify specific entities, like product names, prices, reviews, etc., from web pages and extract the information systematically to enable analytics.
This helps businesses compare the products of multiple competitors on key parameters and make informed decisions. To derive valuable insights into customer preferences and understand how they feel about the products in question, sentiment analysis can be applied to the scraped data.
Multiformat Data Insights: Using Vision AI techniques for web scraping automates the extraction and analysis of visual content like images and videos. Custom vision models can be built and trained for a variety of use cases. They can recognize and classify images, extract text from photos using OCR, detect objects and sentiments within visuals, and perform visual searches. These capabilities enable more comprehensive data collection and deeper insights.
Suppose, you are an e-commerce company wanting to aggregate a product catalog after careful competitor analysis. You can use Vision AI-enabled tools to scrape multiple e-commerce websites and extract product images, analyze them for attributes like color, style, and brand logo recognition, and gather pricing information. This thorough data collection and analysis helps you make informed strategic decisions.
Cost and Time-Saving Solutions: Custom-built intuitive AI data scraping platforms come with easily understandable dashboards and reports, and their workflows and operations are simple. So, you need not employ data specialists or coders. Your non-technical staff can do the job effectively. This helps optimize your research and resource budgets bringing cost efficiencies.
Besides, AI-powered scraping tools save time by processing data much faster. They adapt to different web environments to carry out the scraping process effectively. These tools handle huge volumes of data accurately and automate operations. This helps your resources working on data mining projects improve outcomes by focusing on strategic thinking and creativity.
Scalable Data Harvesting: As AI brings adaptive scraping techniques and human-like browsing patterns, provides multiformat data insights, and improves the quality of outcomes while saving time and cost, it improves the scalability of data harvesting manyfold.
Custom-built fine-grained AI models can manage user-specified tasks like analyzing competitor pricing with precision. AI algorithms can crawl hundreds of web pages simultaneously while applying the extraction logic to complete user-defined tasks. AI helps reduce human intervention by automating the rule-creation process and streamlining the data extraction processes, resulting in high scalability.
AI & Web Scraping: The Future of Data Extraction
As discussed, AI integration is the latest evolution in the history of web scrapers. As technology advances, AI-driven data harvesting will witness more automation, better accuracy, and deeper insights. Businesses will continue to identify and grab opportunities with data-driven decisions. However, it is critical to consider ethical scraping practices and comply with legal and ethical standards. So, you need custom web scraping solutions driven by the latest techniques and best practices.
We specialize in building personalized Artificial Intelligence Solutions to solve our client's issues. We can help you attain flexible, efficient, and accurate data collection processes by using Generative AI.
Explore our case study in the fishing industry, where we combined Gen AI techniques and Web Scraping to automate the processes of extracting, structuring, and storing desired data. We built a mechanism to ensure the processes repeat periodically and maintain the most updated state of data in the database at all times.
We’ve partnered with clients across many industries to build personalized solutions concerning their data collection issues. Let’s schedule a call and discuss how we can help you!
Need web scrapers integrating advanced AI techniques?
We have the skills, experience, and expertise in building high-performing Artificial Intelligence solutions.